Gorimar On The 'Big Pharma Tilt' Towards AI
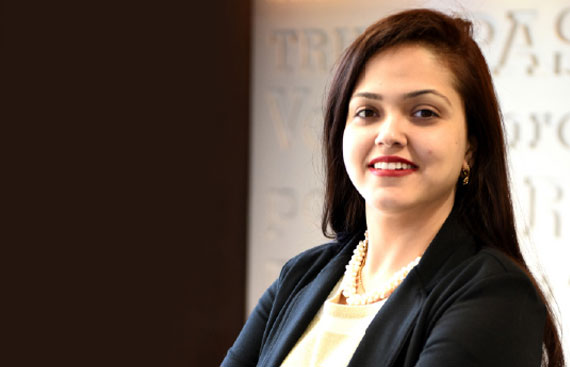
The world’s leading drug companies are turning to artificial intelligence to improve their business of finding new drugs and medicines. These Big Pharmas aim to harness modern technology and machine learning systems to further predict how molecules will behave and the likeliness to make a successful drug. This would not only save them time but also money on unnecessary tests.
“Intelligence” can be defined as the use of past experiences or data to predict the future in a way that proves beneficial. Human intelligence is built upon analogic thought, while more artificial intelligence is based on datasets of multiple experiences. AI systems already play a central role in other high-tech areas. The Big Pharma’s are starting to exploit this potential and how it can help improve efficiencies.
However, when it comes to AI, big pharma is treading cautiously, fully aware that the technology has yet to demonstrate it can successfully bring a new molecule from computer screen to lab to clinic and finally to market. In most drug companies, the system is built to deliver decision support information up the ladder to help senior managers make decisions and apply resources.
A lot of the present use of AI is found in drug discovery and drug development. Other AI tools are used to predict the appropriateness of a given patient for a clinical trial, by using any number of criteria and different datasets from medical records to physical examination to radiology work-up. Other evolving fine-tuned applications that apply AI include precision medicine and predictive medicine - applications where treatment protocols are predicted for a given patient rather than for a group. The business applications of AI include, treasury management to customer service using bots to proper adverse event detection and reporting directly to the FDA.
Key factors to consider when evaluating AI for a specific function:
Senior Leadership Support
Definition of the ‘problem statement’ - key inputs and expected outputs that will support a cost v/s benefit comparison
Data availability for AI to use – clean data in large datasets
Skill set - Know your end user working with AI.
An interesting area in AI is also the use of ‘deep learning’ to predict in-market observations of a drug’s performance in a clinical population. This can help pharmas truly understand drug performance at a market level. Deep learning can often detect subtle ‘conditional information’ that does not have a classification or label until studied. Pharmas do not always understand these ‘subtle effects/interactions’ found in drug populations. Additionally, Pharmas have very little incentive to document this -although there are benefits and other reasons for the public/ consumer population to intelligently understand drug effects. This difference in public interest versus business interest needs to be balanced carefully. Sometimes pharmas give up very good drugs because they do not yet have sensitive mechanisms to maintain these studies and further propagate them. Big Data and AI might allow the intelligent use of drugs that are probably considered not useful when simply released for all customers.
Nevertheless, many big pharma companies are now beginning to recognize that AI, in its own right, represents a burgeoning, new commercial opportunity. The Pharmaceutical industry does invariably have a social obligation to reduce the cost of drugs and reduce the time it takes to get medicines to patients and into the market.