AI enables retailers achieve the Holy Grail of e-Commerce: Arish Ali
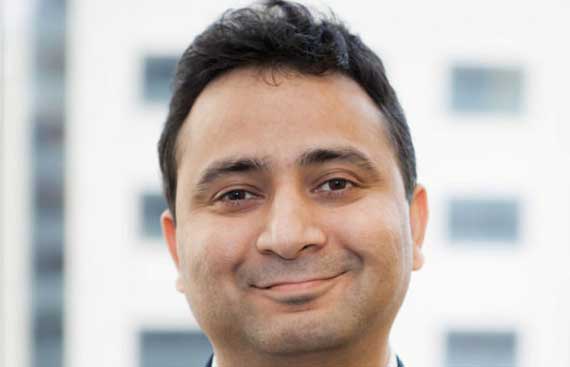
Imagine a retail store which only carries items that you want - including those that you walked in the store to buy as well as those you did not know about but now, having seen them, you are compelled to buy. That is the Holy Grail of e-commerce.
If this could be achieved, the shopping experience for consumers would be more enjoyable and effective. This would also help retailers be more efficient in merchandize selection and planning, resulting in significant cost savings.
Artificial Intelligence (AI) has finally matured to a level where it can help retailers achieve this Holy Grail of e-commerce. AI can reshape customer journey, enabling brands and service providers to deliver personalized, engaging experiences. AI systems powered by machine and statistical learning as well as other sophisticated methods are essentially mechanisms that can delve through structured and unstructured data and infer meaningful insights. Many such systems have taken a step further not only making impressive inferences from the data but also using them to improve the very algorithms that make these inferences.
Personalising shopping experience with AI:
In e-commerce, such systems can tell retailers a lot about their consumers: classify them into effective segments and tiers, and make reliable predictions as to the actions and preferences these consumers are considering.
Both machine learning and statistical learning make use of multitudes of parameters (referred to as “features”), often times up to several hundreds of thousands, to learn from and make inferences about customers’ future behaviour. “Customers who bought this may also be interested in that” is one such example. With deep learning and true AI algorithms, more effective recommendations can be provided. Similarly, by realizing patterns early in the customer journey, retailers can effectively segment customers and offer a personalized shopping experience not only to various user segments but also to individual customers. These shoppable experiences are not limited to tailored products and offerings, special pricing and promotions and powerful recommendations. Even the most esoteric piece of datum, both structured and unstructured may prove relevant in improving these predictions and segmentations. With the commoditization and plummeting costs of storage, retailers hang onto every piece of datum about their shoppers they can put their hands on.
AI is interwoven into every touch point - mPOS, kiosks, mobile apps as well as inventory management where an estimating optimal inventory level is key. In recent years we have seen a paradigm shift into more sophisticated and complex commerce scenarios. Historically, shoppers bought mostly for themselves and interfaced with the store associates or virtual associates via online chats. Today, customers are often buy for others, maintain wish lists, and are heavily influenced by social media. Brands are therefore tasked with more complex tasks, such as real-time sentiment analysis, to prioritize certain offerings and address gaps in their brand equity. Furthermore, the proverbial turing test where one is asked to determine whether one is conversing with a real person or AI is no longer science fiction. Effective chatbots where customers are assisted by bots are now common. Brands can thus provide a follow-the-sun and around-the-clock cheaper and, in many cases, an effective customer support. Harnessing the power of big data, such AI driven recommendations and observations are surprisingly more accurate and relevant than human ones, resulting in the ultimate personalized shopping experience.
AI has not only redefined digital application and customer interfacing touch points, but also back-end capabilities and systems. Consider for example the use case of AI augmented marketing campaigns and automation. AI can infer from past campaigns as well as observable data whose content is more likely to be read, propose customer segments, segmentation parameters, and make recommendations on real-time pricing and promotions. Such tasks are too complex to be executed as effectively by humans alone, involving vast amounts of data to make informed decisions from. Moreover, with the advent of IoT where data are collected from more sources (e.g. RFID and beacons) than ever before, AI helps triage these data and add vital details to the customer’s profile, which in turn, improves the quality of the recommendations and predictions.
Key trends to look forward to:
A key trend to note is the “democratization of AI”. If implementation of AI has traditionally called for well-trained engineers, we start to see cases where non-technical teams and citizen developers are actively looking for ways to utilize AI with minimal assistance from IT. This trend will most likely increase as retailers look to remove yet another barrier between business teams and customers.
AI is a pivotal ingredient and strategic factor in future ready e-commerce solutions. Such systems must not only enhance their front-end and back-end e-commerce capabilities with AI, but also take a step further and empower non-technical teams with the ability to utilize such capabilities effectively, with minimal assistance from IT. This is not a trivial endeavour. Most systems today fall short from this mark. Folding in AI capabilities effectively is not only a major differentiator but also a score point. It is yet another factor to shed light on the decision of many large retailers to build their in-house e-commerce solution.