5 Key Elements for Machine Learning to Detect Fraudsters
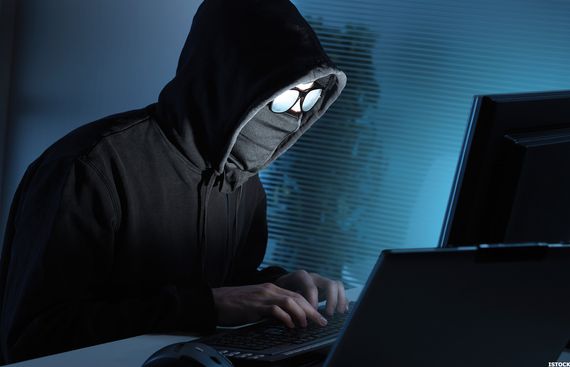
Detecting fraud is quite a daunting task, but within an organization, a very small percentage of activity of fraud can turn into a huge loss of amount if the right tools and systems are not used. With a sophisticated machine learning system, organizations can safely stay one step ahead of fraudsters, as they are constantly evolving and adapting new techniques to commit. To fight fraud, the best technology for fighting fraud is the one that can evolve and adapt rapidly according to fraudsters’ tactics. However, there are five components that are required to apply Machine Learning (ML) to detect fraud.
1. Diversity
A single algorithm or method cannot work best for fraud detection. Testing, evaluating and analyzing different methods to detect fraud leads to success. With the help of a toolkit and a variety of supervised and unsupervised method, ML can be effective. Using engineering techniques, ML requires a toolkit, which can quickly adapt and improve.
2. Quality Data
Data sets are growing daily, and ML applications need that quality set of data to build anti-fraud ML systems. As data grows, it becomes difficult to detect fraud and different methods can be tried to test this. Hence, more is the data, more are the chances to test and build a system. It is always recommended to develop an ML platform that can scale the data and complexity increase.
3. Integration
Reports state that, only 50 percent of the models developed will ever make it into production, and it shows the effort wasted in developing. Developing, deploying and running is never an easy task and as of now, deploying a model in an operational run-time environment is the challenge. The key is the portability of the model and integration of the decision logic within operational systems to detect fraud at scale.
4. Interpretability
Generally, it is very difficult to explain to decision-makers how the model came to score. ML methods and models are mostly black boxes. Explaining ‘what’ and ‘how’ for the ML systems is crucial, especially in the financial services. Interpretability is very vital as it needs for supporting model validation and governance processes. Often, this explaining process is termed as ‘white-boxing’.
5. Monitoring and Experimenting
For most ML fraud detecting systems, the ongoing monitoring is essential as data and population increases in a rapid pace. The increasing demands for a system upgrade, can improve the overall performance in a great deal. As fraudsters bring new unidentified patterns and changes, these ML methods can eliminate the possibility.
Organizations are now investing heavily on the data scientists who can freely experiment on the ML models, as technology changes every day. Data scientists can freely experiment with a variety of methods, data and techniques to get rid of the fraud trends.
Read More News :
APM Means Online, Speed and Security, Says Prince Joseph
Sustain Performance and User Experience through APM Tech: Amit Pal Singh